Generation of Realistic Gene Regulatory Networks by Enriching for Feed-Forward Loops
The regulatory relationships between genes and proteins in a cell form a gene regulatory network (GRN) that controls the cellular response to changes in the environment. A number of inference methods to reverse engineer the original GRN from large-scale expression data have recently been developed. However, the absence of ground-truth GRNs when evaluating the performance makes realistic simulations of GRNs necessary. One aspect of this is that local network motif analysis of real GRNs indicates that the feed-forward loop (FFL) is significantly enriched. To simulate this properly, we developed a novel motif-based preferential attachment algorithm, FFLatt, which outperformed the popular GeneNetWeaver network generation tool in reproducing the FFL motif occurrence observed in literature-based biological GRNs. It also preserves important topological properties such as scale-free topology, sparsity, and average in/out-degree per node. We conclude that FFLatt is well-suited as a network generation module for a benchmarking framework with the aim to provide fair and robust performance evaluation of GRN inference methods.
CITE THIS COLLECTION
REFERENCES
- https://doi.org//10.3389/fbioe.2015.00157
- https://doi.org//10.1016/j.nancom.2015.04.002
- https://doi.org//10.1098/rsif.2016.0179
- https://doi.org//10.1103/PhysRevLett.114.088101
- https://doi.org//10.1038/s41592-021-01252-x
- https://doi.org//10.1016/j.jmb.2006.04.029
- https://doi.org//10.1126/science.286.5439.509
- https://doi.org//10.1073/pnas.0400087101
- https://doi.org//10.1126/science.aad9029
- https://doi.org//10.1016/j.cell.2005.08.020
- https://doi.org//10.1186/s12859-018-2217-z
- https://doi.org//10.1186/s12859-016-1040-7
- https://doi.org//10.1038/nature09645
- https://doi.org//10.3390/make1010008
SHARE
Usage metrics
Read the peer-reviewed publication
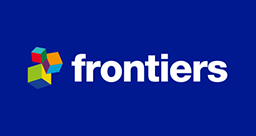
AUTHORS (4)
- EZErik K. ZhivkopliasOVOleg VavulovTHThomas HillertonESErik L. L. Sonnhammer
CATEGORIES
- Gene and Molecular Therapy
- Gene Expression (incl. Microarray and other genome-wide approaches)
- Genetics
- Genetically Modified Animals
- Livestock Cloning
- Developmental Genetics (incl. Sex Determination)
- Epigenetics (incl. Genome Methylation and Epigenomics)
- Biomarkers
- Genomics
- Genome Structure and Regulation
- Genetic Engineering