From genes to drugs: targeting Alzheimer’s with circadian insights
Alzheimer’s disease (AD) is a typical neurodegenerative disease that presents challenges due to the lack of biomarkers to identify AD. A growing body of evidence highlights the critical role of circadian rhythms in AD.
MethodsThe differentially expressed clock genes (DECGs) were identified between AD and ND groups (non-demented controls). Functional enrichment analysis was executed on the DECGs. Candidate diagnostic biomarkers for AD were screened by machine learning. ROC and nomograms were constructed to evaluate candidate biomarkers. In addition, therapeutics targeting predictive biomarkers were screened through the DGIdb website. Finally, the mRNA–miRNA network was constructed.
ResultsNine genes were identified through the DECG analysis between the AD and ND groups. Enrichment analysis of nine genes indicated that the pathways were enriched in long-term potentiation and circadian entrainment. Four clock genes (GSTM3, ERC2, PRKCG, and HLA-DMA) of AD were screened using Lasso regression, random forest, SVM, and GMM. The diagnostic performance of four genes was evaluated by the ROC curve. Furthermore, the nomogram indicated that ERC2, PRKCG, and HLA-DMA are good biomarkers in diagnosing AD. Single-gene GSEA indicated that the main enrichment pathways were oxidative phosphorylation, pathways of neurodegeneration-multiple diseases, etc. The results of immune cell infiltration analysis indicated that there were significant differences in 15 immune cell subsets between AD and ND groups. Moreover, 23 drugs targeting HLA-DMA and 8 drugs targeting PRKCG were identified through the DGIdb website.
ConclusionWe identified three predictive biomarkers for AD associated with clock genes, thus providing promising therapeutic targets for AD.
CITE THIS COLLECTION
REFERENCES
- https://doi.org//10.1016/j.pnpbp.2023.110721
- https://doi.org//10.1007/s11357-021-00477-0
- https://doi.org//10.1016/j.nbd.2019.104507
- https://doi.org//10.1016/j.neuroscience.2024.08.008
- https://doi.org//10.1186/s13195-023-01202-z
- https://doi.org//10.24272/j.issn.2095-8137.2022.289
- https://doi.org//10.1146/annurev-biochem-060308-103103
- https://doi.org//10.3389/fimmu.2023.1182307
- https://doi.org//10.1126/science.aav2546
- https://doi.org//10.1016/j.biopsych.2023.10.008
- https://doi.org//10.1038/s41598-023-46560-8
- https://doi.org//10.1126/science.1180962
- https://doi.org//10.1186/s40035-022-00296-z
- https://doi.org//10.1126/scitranslmed.aax3519
SHARE
Usage metrics
Read the peer-reviewed publication
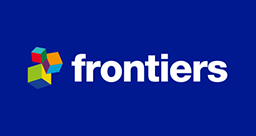
AUTHORS (8)
- ZLZekun LiXLXiaohan LiLSLei SuZZZibo ZhangHGHongmin GuoYGYihao GeFDFang DongFZFeng Zhang