Data from Radiomic Detection of EGFR Mutations in NSCLC
Radiomics is defined as the use of automated or semi-automated post-processing and analysis of multiple features derived from imaging exams. Extracted features might generate models able to predict the molecular profile of solid tumors. The aim of this study was to develop a predictive algorithm to define the mutational status of EGFR in treatment-naïve patients with advanced non–small cell lung cancer (NSCLC). CT scans from 109 treatment-naïve patients with NSCLC (21 EGFR-mutant and 88 EGFR-wild type) underwent radiomics analysis to develop a machine learning model able to recognize EGFR-mutant from EGFR-WT patients via CT scans. A “test–retest” approach was used to identify stable radiomics features. The accuracy of the model was tested on an external validation set from another institution and on a dataset from the Cancer Imaging Archive (TCIA). The machine learning model that considered both radiomic and clinical features (gender and smoking status) reached a diagnostic accuracy of 88.1% in our dataset with an AUC at the ROC curve of 0.85, whereas the accuracy values in the datasets from TCIA and the external institution were 76.6% and 83.3%, respectively. Furthermore, 17 distinct radiomics features detected at baseline CT scan were associated with subsequent development of T790M during treatment with an EGFR inhibitor. In conclusion, our machine learning model was able to identify EGFR-mutant patients in multiple validation sets with globally good accuracy, especially after data optimization. More comprehensive training sets might result in further improvement of radiomics-based algorithms.
Significance:These findings demonstrate that data normalization and “test–retest” methods might improve the performance of machine learning models on radiomics images and increase their reliability when used on external validation datasets.
CITE THIS COLLECTION
FUNDING
Italian Ministry of Health
San Paolo Company
SHARE
Usage metrics
Read the peer-reviewed publication
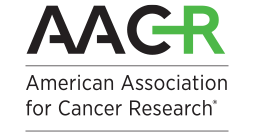
AUTHORS (18)
- GRGiovanni RossiEBEmanuele BarabinoAFAlessandro FedeliGFGianluca FicarraSCSimona CocoARAlessandro RussoVAVincenzo AdamoFBFrancesco BuemiLZLodovica ZulloMDMariella DonoGDGiuseppa De LucaLLLuca LongoMDMaria Giovanna Dal BelloMTMarco TagliamentoAAAngela AlamaGCGiuseppe CittadiniPPPaolo PronzatoCGCarlo Genova